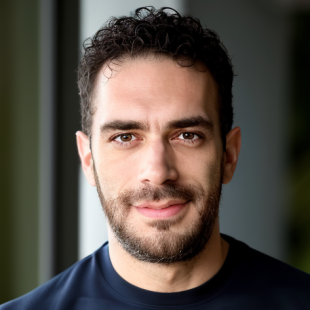
Matthew Caron, M.Sc.
Wirtschaftsinformatik, insb. Data Analytics
Wissenschaftlicher Mitarbeiter
Externer Doktorand
- E-Mail:
- matthew.caron@uni-paderborn.de
Publikationen
Ausgewählte Publikationen
P. Dieter, M. Caron, G. Schryen, European Journal of Operational Research (EJOR) 311 (2023) 283–300.
M. Caron, in: 2022 IEEE International Conference on Big Data (Big Data), IEEE, 2022.
O. Müller, M. Caron, M. Döring, T. Heuwinkel, J. Baumeister, in: 8th Workshop on Machine Learning and Data Mining for Sports Analytics (ECML PKDD 2021), n.d.
M. Caron, M. Gulenko, O. Müller, in: 42nd International Conference on Information Systems (ICIS 2021), 2021.
M. Caron, O. Müller, in: 2020 IEEE International Conference on Big Data (Big Data), 2020, pp. 4383–4391.
Alle Publikationen anzeigen