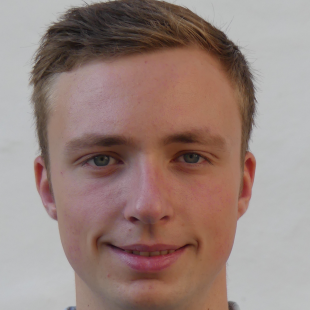
- E-Mail:
- sven.huesing@uni-paderborn.de
- Telefon:
- +49 5251 60-6614
- Büroanschrift:
-
Fürstenallee 11
33102 Paderborn - Raum:
- F2.201
Forschung
Publikationen
Aktuelle Publikationen
Using Worked Examples for Engaging in Epistemic Programming Projects
S. Hüsing, C. Schulte, S. Sparmann, M. Bolte, in: Proceedings of the 55th ACM Technical Symposium on Computer Science Education V. 1, ACM, 2024.
Identifying K-12 Students' Approaches to Using Worked Examples for Epistemic Programming
S. Hüsing, S. Sparmann, C. Schulte, M. Bolte, in: Proceedings of the 2024 Symposium on Eye Tracking Research and Applications, ACM, 2024.
Epistemic Programming
S. Hüsing, C. Schulte, F. Winkelnkemper, in: Computer Science Education, Bloomsbury Academic, 2023.
JuGaze: A Cell-based Eye Tracking and Logging Tool for Jupyter Notebooks
S. Sparmann, S. Hüsing, C. Schulte, in: Proceedings of the 23rd Koli Calling International Conference on Computing Education Research, ACM, 2023.
A PLACE FOR A DATA SCIENCE PROJECT IN SCHOOL: BETWEEN STATISTICS AND EPISTEMIC PROGRAMMING
S. PODWORNY, S. Hüsing, C. SCHULTE, STATISTICS EDUCATION RESEARCH JOURNAL 21 (2022).
Alle Publikationen anzeigen