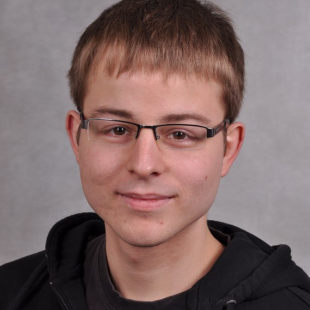
Christoph Böddeker
Nachrichtentechnik (NT) / Heinz Nixdorf Institut
Wissenschaftlicher Mitarbeiter
Forschung & Lehre
- E-Mail:
- boeddeker@nt.uni-paderborn.de
- Telefon:
- +49 5251 60-5288
- Web:
- Homepage
- Büroanschrift:
-
Pohlweg 47-49
33098 Paderborn - Raum:
- P7.2.06
Publikationen
Aktuelle Publikationen
C. Boeddeker, A.S. Subramanian, G. Wichern, R. Haeb-Umbach, J. Le Roux, IEEE/ACM Transactions on Audio, Speech, and Language Processing 32 (2024) 1185–1197.
T. Cord-Landwehr, C. Boeddeker, C. Zorilă, R. Doddipatla, R. Haeb-Umbach, in: ICASSP 2024 - 2024 IEEE International Conference on Acoustics, Speech and Signal Processing (ICASSP), IEEE, 2024.
T. Cord-Landwehr, C. Boeddeker, C. Zorilă, R. Doddipatla, R. Haeb-Umbach, in: ICASSP 2023 - 2023 IEEE International Conference on Acoustics, Speech and Signal Processing (ICASSP), IEEE, 2023.
T. Cord-Landwehr, C. Boeddeker, C. Zorilă, R. Doddipatla, R. Haeb-Umbach, in: INTERSPEECH 2023, ISCA, 2023.
R. Aralikatti, C. Boeddeker, G. Wichern, A. Subramanian, J. Le Roux, in: ICASSP 2023 - 2023 IEEE International Conference on Acoustics, Speech and Signal Processing (ICASSP), IEEE, 2023.
Alle Publikationen anzeigen