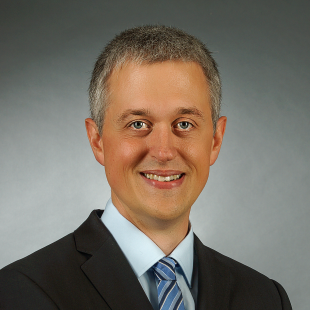
Dr.-Ing. Jörg Schmalenströer
Nachrichtentechnik (NT) / Heinz Nixdorf Institut
Akademischer Oberrat
(Auftrags-)Forschung & Lehre
- E-Mail:
- schmalen@nt.uni-paderborn.de
- Telefon:
- +49 5251 60-3623
- Web:
- Homepage
- Büroanschrift:
-
Pohlweg 47-49
33098 Paderborn - Raum:
- P7.2.05
Publikationen
Aktuelle Publikationen
T. Gburrek, J. Schmalenstroeer, R. Haeb-Umbach, in: European Signal Processing Conference (EUSIPCO), 2023.
J. Schmalenstroeer, T. Gburrek, R. Haeb-Umbach, in: ITG Conference on Speech Communication, 2023.
T. Gburrek, J. Schmalenstroeer, R. Haeb-Umbach, in: Proc. Asilomar Conference on Signals, Systems, and Computers, 2023.
J. Heitkämper, J. Schmalenstroeer, R. Haeb-Umbach, in: Proceedings of the 30th European Signal Processing Conference (EUSIPCO), Belgrad, n.d.
H. Afifi, H. Karl, T. Gburrek, J. Schmalenstroeer, in: 2022 International Wireless Communications and Mobile Computing (IWCMC), IEEE, 2022.
Alle Publikationen anzeigen
Lehre
Laufende Lehrveranstaltungen
- Zeitdiskrete Signalverarbeitung
- Nachrichtentechnik (Projekt)
- Digitale Sprachsignalverarbeitung
- Digital Speech Signal Processing
- Deep Learning (Projekt)
- Deep Learning (Project)
- Communications and Speech Processing (Project)
- Communications Engineering (Project)
Weitere Informationen
Honors & Distinctions
- 2013: Forschungspreis der Universität Paderborn: Verlässliche Navigation in Gebäuden
- 2017: "Top 10% Paper Award" at IEEE Multimedia Signal Processing Workshop, Luton, U.K.. Paper title: Multi-Stage Coherence Drift Based Sampling Rate Synchronization for Acoustic Beamforming, by. J. Schmalenstroeer, J. Heymann, L. Drude, C. Boeddeker, and R. Haeb-Umbach
Projects
- Verlässliche Navigation in Gebäuden
2013 - 2014
Forschungspreis der Universität Paderborn - Bonuspoint: Entwicklung eines Algorithmus zur Positionsbestimmung für das Fujitsu Forum 2014
Oct. 2014 - Dec. 2014
Fujitsu Technology Solutions GmbH - Product Development Group / Innovation Lab - Big Data Real Time Shopping Assistant: Entwicklung eines Client-Server-basierten Ansatzes zur Positionsbestimmung mittels Bluetooth Low Energy
Mar. 2015 - Dec. 2015
Fujitsu Technology Solutions GmbH - Product Development Group / Innovation Lab - Entwicklung einer Bluetooth Low Energy Kommunikationsplattform
Nov. 2015 - Nov. 2016
Hörmann KG Antriebstechnik - Verteilte akustische Signalverarbeitung über funkbasierte Sensornetzwerke
2016 - 2019
Deutsche Forschungsgemeinschaft Forschungsgruppe: DFG FOR 2457 "Akustische Sensornetzwerke"