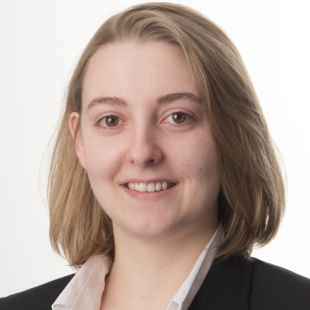
Tanja Tornede
Mitglied - Ehemalige
- E-Mail:
- t.tornede@ai.uni-hannover.de
- Web:
- Homepage
- Sprechstunden:
Im Februar 2023 habe ich die Universität Paderborn verlassen. Zu finden bin ich nun woanders.
Publikationen
Aktuelle Publikationen
A. Tornede, L. Gehring, T. Tornede, M.D. Wever, E. Hüllermeier, Machine Learning (2022).
B. Hammer, E. Hüllermeier, V. Lohweg, A. Schneider, W. Schenck, U. Kuhl, M. Braun, A. Pfeifer, C.-A. Holst, M. Schmidt, G. Schomaker, T. Tornede, Schlussbericht ITS.ML: Intelligente Technische Systeme der nächsten Generation durch Maschinelles Lernen. Forschungsvorhaben zur automatisierten Analyse von Daten mittels Maschinellen Lernens, 2022.
T. Tornede, A. Tornede, M.D. Wever, E. Hüllermeier, in: Proceedings of the Genetic and Evolutionary Computation Conference, 2021.
T. Tornede, A. Tornede, J.M. Hanselle, M.D. Wever, F. Mohr, E. Hüllermeier, ArXiv:2111.05850 (2021).
T. Tornede, A. Tornede, M.D. Wever, F. Mohr, E. Hüllermeier, in: Proceedings of the ECMLPKDD 2020, 2020.
Alle Publikationen anzeigen
Weitere Informationen
New Position
I left Paderborn University in February 2023.