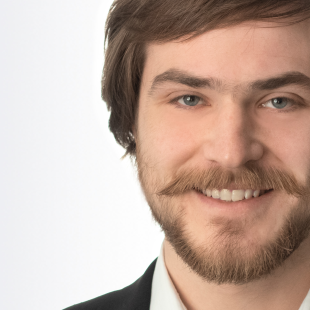
Stefan Heid, M.Sc.
- E-Mail:
- stefan.heid@lmu.de
- Büroanschrift:
-
Zukunftsmeile 2
33102 Paderborn - Raum:
- ZM2.B.02.27
Ehemaliger
Ehemaliger
- E-Mail:
- stefan.heid@uni-paderborn.de
- Büroanschrift:
-
Zukunftsmeile 2
33102 Paderborn - Raum:
- ZM2.A.01.02-1
Publikationen
Aktuelle Publikationen
Learning decision catalogues for situated decision making: The case of scoring systems
S. Heid, J.M. Hanselle, J. Fürnkranz, E. Hüllermeier, International Journal of Approximate Reasoning 171 (2024).
Learning decision catalogues for situated decision making: The case of scoring systems
S. Heid, J.M. Hanselle, J. Fürnkranz, E. Hüllermeier, International Journal of Approximate Reasoning 171 (2024).
Safe Bayesian Optimization for Data-Driven Power Electronics Control Design in Microgrids: From Simulations to Real-World Experiments
D. Weber, S. Heid, H. Bode, J. Lange, E. Hüllermeier, O. Wallscheid, IEEE Access 9 (2021) 35654–35669.
Towards a Scalable and Flexible Simulation and Testing Environment Toolbox for Intelligent Microgrid Control
H. Bode, S.H. Heid, D. Weber, E. Hüllermeier, O. Wallscheid, ArXiv:2005.04869 (2020).
Constrained Multi-Agent Optimization with Unbounded Information Delay
S.H. Heid, A. Ramaswamy, E. Hüllermeier, in: Proceedings-30. Workshop Computational Intelligence: Berlin, 26.-27. November 2020, 2020, p. 247.
Alle Publikationen anzeigen