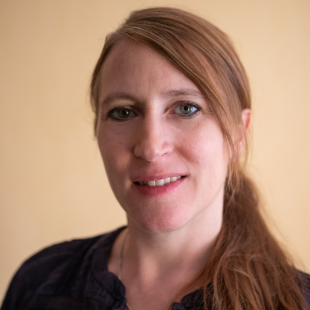
Prof. Dr. Sina Ober-Blöbaum
- E-Mail:
- sinaober@math.uni-paderborn.de
- Büroanschrift:
-
Technologiepark 21
33100 Paderborn - Raum:
- TP21.1.12
Über Sina Ober-Blöbaum
Curriculum Vitae
Seit 2020: Professorin (W3)
Universität Paderborn
12/2021 - 12/2022: Elternzeit
2015 - 2020: Außerordentliche Professorin
Universität Oxford, UK
10/2018 - 06/2019: Elternzeit
01/2017 - 08/2017: Elternzeit
2014 - 2015: Gastprofessorin
Freie Universität Berlin, Deutschland
2009 - 2015: Juniorprofessorin
Universität Paderborn
2013 - 2014: Gastprofessorin
Technische Universität Dresden, Deutschland
2011 - 2012: Vertretungsprofessorin
Technische Universität München, Deutschland
2008 - 2009: Postdoc
California Institute of Technology, Pasadena, California, USA
13.03.2008: Promotion
Angewandte Mathematik, Universität Paderborn, Deutschland. Betreuer: Prof. Dr. Michael Dellnitz
1999 - 2004: Studium
Diplom in Technomathematik, Universität Paderborn
Seit 2016: Ausgewählte Aufgaben auf Einladung
2021: Conference on the Numerical Solution of Differential and Differential-Algebraic Equations (NUMDIFF-16, Halle, Deutschland, 2021), Plenarvortrag
2016: 4th European Conference on Computational Optimization (EUCCCO, Leuven, Belgien, 2016), Plenarvortrag
2018: Oberwolfach Research in Pairs, Genehmigung eines zweiwöchigen Forschungsaufenthalts am Mathematischen Forschungsinstitut Oberwolfach, Deutschland
2011 - 2014: Mitglied des "Jungen Kollegs" der Nordrhein-Westfälischen Akademie der Wissenschaften und der Künste, Deutschland
2013: "Eleonore-Trefftz-Gastprofessur" an der Technischen Universität Dresden, Deutschland
2011: Best Paper Award, 8th Paderborn Workshop on Design of mechatronic systems, Paderborn, Deutschland
2011: Best Poster Award, International Conference on Simulation Technology, Stuttgart, Deutschland
2009: Preis für die beste Doktorarbeit 2008 der Universität Paderborn
2008: Best Poster Award, Oberwolfach-Seminar "Angewandte Dynamik und Geometrische Mechanik", Oberwolfacher Forschungsinstitut für Mathematik, Deutschland
Forschung
Forschungsschwerpunkte
- strukturerhaltende Integration
- Lagrange- und Hamiltonsysteme und Variationsintegratoren
- fraktionale und dissipative Lagrangesysteme
- Multiratensysteme und -integratoren
- numerische Verfahren in der Optimalen Steuerung
- Symmetrien und Turnpike-Eigenschaften in der optimalen Steuerung
- Lernen von Hamilton- und Lagrangesystemen
- Anwendungen in Astrodynamik, Antriebstechnik und Quantendynamik
Ausgewählte Projekte
- Symplektische Diskretisierungen für Optimalsteuerungsprobleme mechanischer Systeme
- PhoQC: Photonisches Quantencomputing
- Fractional Variational Integration and Optimal Control
- Intelligente technische Systeme OWL, Querschnittsprojekt Selbstoptimierung
- SFB 614 - Entwicklung und Anwendung von Methoden zur Mehrzieloptimierung und optimalen Steuerung, sowie zur Berechnung dynamischen Verhaltens hybrider Systeme (Teilprojekt A01)
Publikationen
Ausgewählte Publikationen
T. Faulwasser, K. Flaßkamp, S. Ober-Blöbaum, M. Schaller, K. Worthmann, Mathematics of Control, Signals, and Systems 34 (2022) 759–788.
C. Offen, S. Ober-Blöbaum, Chaos: An Interdisciplinary Journal of Nonlinear Science 32(1) (2022).
F. Jiménez, S. Ober-Blöbaum, in: Nichtlineare Sci 31, 2021.
D.J.N. Limebeer, S. Ober-Blöbaum, F.H. Farshi, IEEE Transactions on Automatic Control 65(4) (2020) 1381–1396.
S. Ober-Blöbaum, IMA Journal of Numerical Analysis 37(1) (2017) 375–406.
Alle Publikationen anzeigen
Lehre
Laufende Lehrveranstaltungen
- Seminar
- Oberseminar AG Ober-Blöbaum
Wissenschaftliches Engagement
Seit 2011 | Mitglied der wissenschaftlichen Kommissionen von vier internationalen Konferenzen
Seit 2010 | (Mit-)Organisation mehrerer Minisymposien auf internationalen Konferenzen (z. B. ECC, MTNS) und verschiedener Vortragsreihen auf internationalen Sommer-/Winterschulen (z. B. Madrid)
2013 | Organisatorin der Dresdner Mathematischen Reihe "Frauen in der Wissenschaft", TU Dresden, Deutschland
2012 | Organisation des Forschungstages des "Jungen Kollegs" der Nordrhein-Westfälischen Akademie der Wissenschaften und der Künste, Deutschland
Seit 2021 | Mitglied der Steuerungsgruppe von "Frauen gestalten die Informationsgesellschaft" und perspEktIveM (Mentoring-Programm für Studentinnen), Universität Paderborn
2017 - 2020 | Mitglied der beratenden Kommission des DFG-Graduiertenkollegs Pi3: Parameteridentifikation "Analyse, Algorithmen, Implementierungen" der Universität Bremen, Deutschland
2011 - 2015 | Mitglied der Steuerungsgruppe von "Frauen gestalten die Informationsgesellschaft" und perspEktIveM (Mentoring-Programm für Studentinnen), Universität Paderborn
Seit 2017 | Redaktioneller Beirat von AIMS Journal of Computational Dynamics und GEM - International Journal on Geomathematics
2017 | Mitglied im Komitee "Zukunft der GAMM", GAMM (Gesellschaft für Angewandte Mathematik und Mechanik)
Weitere Informationen
Short Bio
I received my Dipl. Math. in 2004 and my Ph.D. degree in applied mathematics in 2008 both from the Paderborn University in Germany. I was a Postdoctoral Scholar at the California Institute of Technology, CA, USA, from 2008 to 2009. From 2009 to 2015 I was a Junior Professor at the Department of Mathematics at the Paderborn University. From 2015 to 2020 I was Associate Professor of control engineering at the Department of Engineering Science and a Tutorial Fellow in Engineering at Harris Manchester College, University of Oxford, Oxford, U.K. Currently, I am full Professor for applied mathematics at the Paderborn University leading the research group "Numerical Mathematics and Control". Our group's research focus lies in the development and analysis of structure-preserving simulation and optimal control methods for mechanical, electrical and hybrid systems, with a wide range of application areas including astrodynamics, drive technology and robotics.