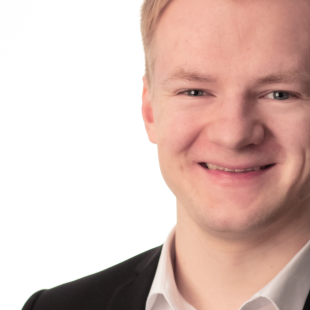
- E-Mail:
- julian.lienen@uni-paderborn.de
- Büroanschrift:
-
Zukunftsmeile 2
33102 Paderborn - Raum:
- ZM2.A.01.05
Publikationen
Aktuelle Publikationen
Detecting Novelties with Empty Classes
S. Uhlemeyer, J. Lienen, E. Hüllermeier, H. Gottschalk, ArXiv:2305.00983 (2023).
Memorization-Dilation: Modeling Neural Collapse Under Noise
D.A. Nguyen, R. Levie, J. Lienen, G. Kutyniok, E. Hüllermeier, in: International Conference on Learning Representations, ICLR, 2023.
Mitigating Label Noise through Data Ambiguation
J. Lienen, E. Hüllermeier, ArXiv:2305.13764 (2023).
Scikit-Weak: A Python Library for Weakly Supervised Machine Learning
A. Campagner, J. Lienen, E. Hüllermeier, D. Ciucci, in: Lecture Notes in Computer Science, Springer, 2022, pp. 57–70.
Kronecker Decomposition for Knowledge Graph Embeddings
C. Demir, J. Lienen, A.-C. Ngonga Ngomo, ArXiv:2205.06560 (2022).
Alle Publikationen anzeigen