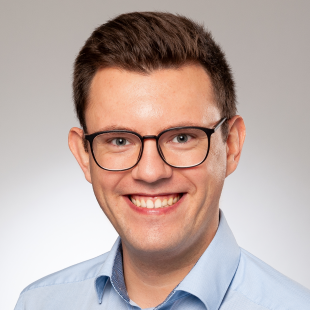
Dr. Alexander Tornede
Mitglied - Ehemaliger
- E-Mail:
- a.tornede@ai.uni-hannover.de
- ORCID:
- 0000-0002-2415-2186
- Web:
- Homepage
- Homepage (Extern)
- Sprechstunden:
Seit September 2022 arbeite ich am Institut für Künstliche Intelligenz an der Leibniz Universität Hannover!
Publikationen
Aktuelle Publikationen
J.M. Hanselle, E. Hüllermeier, F. Mohr, A.-C. Ngonga Ngomo, M. Sherif, A. Tornede, M.D. Wever, in: C.-J. Haake, F. Meyer auf der Heide, M. Platzner, H. Wachsmuth, H. Wehrheim (Eds.), On-The-Fly Computing -- Individualized IT-Services in Dynamic Markets, Heinz Nixdorf Institut, Universität Paderborn, Paderborn, 2023, pp. 85–104.
A. Tornede, Advanced Algorithm Selection with Machine Learning: Handling Large Algorithm Sets, Learning From Censored Data, and Simplyfing Meta Level Decisions, 2023.
J.M. Hanselle, E. Hüllermeier, F. Mohr, A.-C. Ngonga Ngomo, M. Sherif, A. Tornede, M.D. Wever, in: C.-J. Haake, F. Meyer auf der Heide, M. Platzner, H. Wachsmuth, H. Wehrheim (Eds.), On-The-Fly Computing – Individualized IT-Services in Dynamic Markets, Heinz Nixdorf Institut, Universität Paderborn, 2023, pp. 85–104.
E. Schede, J. Brandt, A. Tornede, M.D. Wever, V. Bengs, E. Hüllermeier, K. Tierney, ArXiv:2202.01651 (2022).
L. Fehring, J.M. Hanselle, A. Tornede, in: Workshop on Meta-Learning (MetaLearn 2022) @ NeurIPS 2022, 2022.
Alle Publikationen anzeigen
Weitere Informationen
Neue Position
Seit September 2022 arbeite ich am Institut für Künstliche Intelligenz an der Leibniz Universität Hannover!