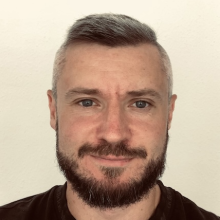
- E-Mail:
- jboss@mail.uni-paderborn.de
- ORCID:
- 0000-0002-4121-4668
- Web:
- Homepage (Extern)
- Social Media:
- Büroanschrift:
-
Fürstenallee 11
33102 Paderborn - Raum:
- FU.307
Über Jakob Bossek
Jakob schloss einen Bachelor-Studium der Statistik sowie ein Diplomstudium der Informatik an der Technischen Universität Dortmund ab. Im Jahr 2018 erhielt er einen Doktorgrad in Information Systems von der Universität Münster. Nach PostDoc-Positionen sowohl an der Universität Münster als auch an der Universität Adelaide (Australien) war er von April 2022 bis November 2023 als Akademischer Rat am Lehrstuhl für AI Methodology (Informatik 14) an der RWTH Aachen tätig. Derzeit ist er Akademischer Rat am Lehrstuhl für Maschinelles Lernen und Optimierung (MALEO) im Fachbereich Informatik an der Universität Paderborn.
Curriculum Vitae
04/2022 - 11/2023: Akademischer Rat an der Fakultät Informatik (Lehrstuhl für Methodik der künstlichen Intelligenz), RWTH Aachen, Deutschland
04/2021 - 03/2022: Akademischer Rat (auf Zeit) am Institut für Wirtschaftsinformatik (Lehrstuhl für Statistik und Optimierung), Universität Münster, Deutschland
09/2020 - 03/2021: PostDoc am Institut für Wirtschaftsinformatik (Lehrstuhl für Statistik und Optimierung), Universität Münster, Deutschland
10/2019 - 09/2020: PostDoc an der School of Computer Science, The University of Adelaide, Australia in der Optimisation and Logistics Gruppe von Prof. Dr. Frank Neumann
02/2015 - 09/2019: Wissenschaftlicher Mitarbeiter (Doktorand bis November 2018; danach PostDoc) am Institut für Wirtschaftsinformatik (Lehrstuhl für Statistik und Optimierung), Universität Münster, Deutschland
11/2018: Erlangung des Doktorgrads in Information Systems (Dr. rer. pol.) an der Universität Münster, Deutschland
09/2005 - 11/2014: Studium der Informatik mit Nebenfach Statistik an der TU-Dortmund (Abschluss: Diplom)
09/2008 - 05/2013: Studium der Statistik mit Nebenfach Informatik an der TU-Dortmund (Abschluss: Bachelor of Science)
If you want truly to understand something, try to change it. - Kurt Levin
Forschung
Forschungsschwerpunkte
Seine Forschungsinteressen umfassen AutoAI-Methoden mit besondererem Fokus auf Auswahl und Konfiguration von Algorithmen (Algorithm Selection bzw. Algorithm Configuration). Sein Hauptforschungsschwerpunkt liegt jedoch auf empirischen sowie theoretischen Aspekten bioinspirierter Optimierung, insbesondere im Bereich evolutionärer Algorithmen für NP-schwere kombinatorische Optimierungsprobleme. Derzeit zielt seine Forschung darauf ab das theoretische Verständnis evolutionärer Algorithmen durch Zeitkomplexitätsanalysen zu vertiefen, insbesondere Quality-Diversity (QD) sowie Evolutionary Diversity Optimisation (EDO) auf kombinatorischen Optimierungsproblemen.
- Mehrkriterielle Optimierung
- Kombinatorische Optimierung
- Heuristische Optimierung
- Evolutionäre Optimierungmethoden
- Theoretische Aspekte randomisierter Suchheuristiken
- AutoAI Methoden (Algorithm Selection and Algorithm Configuration)
- Maschinelles Lernen
- Datenanalyse