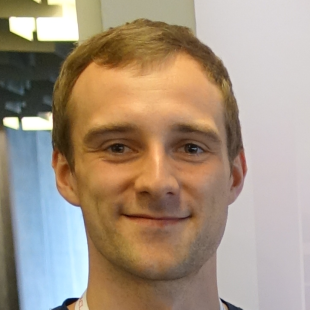
Maximilian Spliethöver
- E-Mail:
- m.spliethoever@ai.uni-hannover.de
- Phone:
- +49 511 762 14998
- Web:
- Homepage (Extern)
Publications
Latest Publications
M. Stahl, M. Spliethöver, H. Wachsmuth, in: Proceedings of the Fifth Workshop on Natural Language Processing and Computational Social Science, Abu Dhabi, United Arab Emirates, n.d.
M. Spliethöver, H. Wachsmuth, in: Proceedings of the Thirtieth International Joint Conference on Artificial Intelligence, IJCAI-21, 2021, pp. 552–559.
M. Alshomary, T. Gurcke, S. Syed, P. Heinisch, M. Spliethöver, P. Cimiano, M. Potthast, H. Wachsmuth, in: Proceedings of the 8th Workshop on Argument Mining, 2021, pp. 184–189.
M. Spliethöver, H. Wachsmuth, in: Proceedings of the 7th Workshop on Argument Mining (ArgMining 2020), 2020, pp. 76–87.
D. Alexandrovsky, G. Volkmar, M. Spliethöver, S. Finke, M. Herrlich, T. Döring, J.D. Smeddinck, R. Malaka, in: Proceedings of the Annual Symposium on Computer-Human Interaction in Play, Association for Computing Machinery, New York, NY, USA, 2020, pp. 32–45.
Show all publications