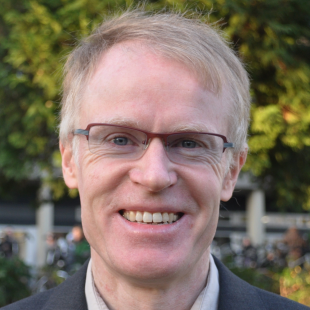
Prof. Dr. Reinhold Häb-Umbach
- E-Mail:
- haeb@nt.uni-paderborn.de
- Phone:
- +49 5251 60-3626
- ORCID:
- 0000-0001-9468-7330
- Web:
- Homepage
- Homepage (Extern)
- Office Address:
-
Pohlweg 47-49
33098 Paderborn - Room:
- P7.2.05.3
- Office hours:
Wed. 16.00h -17.00h
- Office Address:
-
Pohlweg 47-49
33098 Paderborn - Room:
- P7.2.05.3
Research
Research Interests
Statistical signal processing and machine learning for speech and audio applications
Latest Projects
- WestAI - AI Service Center West
- SAIL: SustAInable Life-cycle of Intelligent Socio-Technical Systems
- TRR 318 - Constructing Explainability
- TRR 318 - Technically enabled explanation of speaker traits (Subproject C06)
- Automatic transcription of conversation situations
- Learning deep speech representations for phonetics research
- Explainable Feature Importance: Interpretable machine learning through game theoretic analysis of influence variables and interaction effects
- Technically Enabled Explaining of Speaker Traits
Publications
Latest Publications
C. Boeddeker, A.S. Subramanian, G. Wichern, R. Haeb-Umbach, J. Le Roux, IEEE/ACM Transactions on Audio, Speech, and Language Processing 32 (2024) 1185–1197.
T. Cord-Landwehr, C. Boeddeker, C. Zorilă, R. Doddipatla, R. Haeb-Umbach, in: ICASSP 2024 - 2024 IEEE International Conference on Acoustics, Speech and Signal Processing (ICASSP), IEEE, 2024.
T. Gburrek, J. Schmalenstroeer, R. Haeb-Umbach, in: European Signal Processing Conference (EUSIPCO), 2023.
T. Cord-Landwehr, C. Boeddeker, C. Zorilă, R. Doddipatla, R. Haeb-Umbach, in: ICASSP 2023 - 2023 IEEE International Conference on Acoustics, Speech and Signal Processing (ICASSP), IEEE, 2023.
J. Schmalenstroeer, T. Gburrek, R. Haeb-Umbach, in: ITG Conference on Speech Communication, 2023.
Show all publications
Teaching
Current Courses
- Wireless Communications
- Wireless Communications
- Statistical and Machine Learning
- Statistical and Machine Learning
- Nachrichtentechnik (Projekt)
- Forschungsseminar Nachrichtentechnik
- Deep Learning (Projekt)
- Deep Learning (Project)
- Communications and Speech Processing (Project)
- Communications and Speech Processing (Project)
- Communications Engineering (Project)
Further Information
Honors and Awards
2022
- Co-author of paper winning Best Student Paper Award at IWAENC 2022. Paper title: Monaural Source Separation: From Anechoic to Reverberant Environments, by T. Cord-Landwehr, Christoph Boeddeker, Thilo von Neumann, Catalin Zorila, Rama Doddipatla, Reinhold Haeb-Umbach
2021-2023
- Distinguished Lecturer of the International Speech Communication Association (ISCA).
2020
- Fellow of the Institute of Electrical and Electronics Engineers (IEEE). Denomination: "for contributions to robustness of automatic speech recognition".
2017
- "Top 10% Paper Award" at IEEE Multimedia Signal Processing Workshop, Luton, U.K.. Paper title: Multi-Stage Coherence Drift Based Sampling Rate Synchronization for Acoustic Beamforming, by. J. Schmalenstroeer, J. Heymann, L. Drude, C. Boeddeker, and R. Haeb-Umbach
- Co-author of paper winning the best student paper award at Interspeech 2017, Stockholm, Sweden. Paper title: Hidden Markov Model Variational Autoencoder for Acoustic Unit Discovery, by J. Ebbers, J. Heymann, L. Drude, T. Glarner, R. Haeb-Umbach, and B. Raj
2015
- Fellow of the International Speech Communication Association (ISCA). Denomination: "for contributions to improving robustness of ASR".
2013
- Co-author of paper winning the best student paper award at IEEE Automatic Speech Recognition and Understanding Workshop (ASRU), Olomouc, Czech Republic. Paper title: A Hierarchical System for Word Discovery Exploiting DTW-Based Initialization, by O.Walter, T. Korthals, R. Haeb-Umbach, B. Raj
2012
- Co-author of paper winning the best student paper award at International Workshop on Acoustic Signal Enhancement (IWAENC), Aachen, Germany. Paper title: Exploiting Temporal Correlations in Joint Multichannel Speech Separation and Noise Suppression Using Hidden Markov Models, by D.H. Tran Vu and R. Haeb-Umbach
2011
- Co-author of publication nominated for best student paper Award at Interspeech 2011, Florence, Italy. Paper title: A Versatile Gaussian Splitting Approach to Non-Linear State Estimation and its Application to Noise-Robust ASR, by V. Leutnant, A. Krueger and R. Haeb-Umbach
- Weierstraß-Prize of the University of Paderborn for excellence in teaching
2010
- Co-author of Paper winning the best student paper award at International Workshop on Acoustic Echo and Noise Control (IWAENC), Tel Aviv. Paper title: An EM Approach to Multichannel Speech Separation and Noise Suppression, by D.H. Tran Vu and R. Haeb-Umbach
2009
- Senior Member, IEEE
1997
Innovation Prize of Philips Research for managing a project, which brought the first name-dialing functionality to Philips moble phones
1994
- Innovation Prize of Philips Research for contributions to Philips' Large Vocabulary Continuous Speech Recognition System
1988
- "Borchers Plague" of RWTH Aachen University for dissertation with honours
1983
- "Springorum Plague of RWTH Aachen University for Dipl.-Ing. degree with distinction
1980-1983
- Fellow of "Studienstiftung des Deutschen Volkes" (German Academic Scholarship Foundation)
Professional Highlights
- Co-author of Robust Speech Recognition - A Bridge to Practical Applications, Elsevier, 2016
- Published some 300 papers in peer reviewed journals and conferences
- Co-editors of the book Robust Speech Recognition of Uncertain or Missing Data: Theory and Applications, Springer, 2011
- 15 competitive research grants by DFG (German Research Foundation) in last 15 years
- Currently supervising 7 Ph.D. students
Service to the Scientific Community
- Technical Program Chair, Interspeech 2024
- IEEE Audio and Acoustics Signal Processing Technical Committee Member, 2022 - 2024
- IEEE Speech and Language Processing Technical Committee Member, 2015 – 2020
- Regional Publicity Chair, IEEE Workshop on Spoken Language Technology, Athens, Dec. 2018
- Technical Program Chair, IEEE Automatic Speech Recognition and Understanding Workshop, Okinawa, Japan, Dec. 2017
- Regional Publicity Chair, IEEE Workshop on Spoken Language Technology, San Diego, Dec. 2016
- General Chair, ITG Conference on Speech Communication, October 2016, Paderborn, Germany
- Exhibition Chair of Interspeech 2015, Dresden, Germany
- Chairman of ITG (German Engineering Society) Technical Committee Audiotechnology AT3 on Speech Communication (2016 - 2023); and member of Technical Committee "Signal Processing and Machine Learning"
- Technical Reviewer for journals and conferences, including IEEE Transactions on Audio, Speech and Language Processing, IEEE Signal Processing Magazine, IEEE Signal Processing Letters, Elsevier Speech Communication, Journal of the Acoustical Society of America, Computer Speech and Language, ICASSP, Interspeech, Eusipco, ASRU, SLT, DCASE Workshop, CHiME Workshop, IWAENC
- Reviewer of grant applications to EU, DFG (German Research Foundation) and various foreign research organizations
- Guest editor IEEE Selected Topics in Signal Processing, special issue on "Far-Field Speech Processing in the Era of Deep Learning: Speech Enhancement, Separation and Recognition", vol. 13. no. 4, Aug. 2019
- Organizer of Special Session at ICASSP 2018 on "Multi-Microphone Speech Recognition", Calgary, April 2018
- Guest editor IEEE Selected Topics in Signal Processing, special issue on „Natural Interaction with Intelligent Environments”, vol. 4, no. 5, Oct. 2010