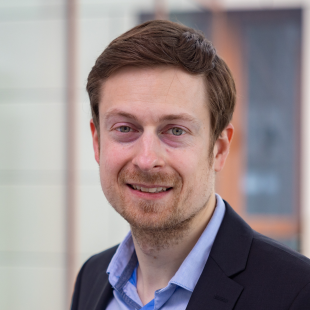
Dr. Stefan Heindorf
Junior Research Group Leader - PostDoc
Junior Research Group Leader Data Science
- E-Mail:
- heindorf@uni-paderborn.de
- ORCID:
- 0000-0002-4525-6865
- Web:
- Homepage
- Social Media:
- Office Address:
-
Fürstenallee 11
33102 Paderborn - Room:
- FU.214
Member - Project Partner
Associated Postdoctoral Researcher
- E-Mail:
- stefan.heindorf@uni-paderborn.de
- Web:
- Homepage
- Homepage (Extern)
- Office Address:
-
Fürstenallee 11
33102 Paderborn - Room:
- FU.214
Publications
Latest Publications
Universal Knowledge Graph Embeddings
N.J. KOUAGOU, C. Demir, H.M.A. Zahera, A. Wilke, S. Heindorf, J. Li, A.-C. Ngonga Ngomo, in: Companion Proceedings of the ACM on Web Conference 2024, ACM, 2024.
Utilizing Description Logics for Global Explanations of Heterogeneous Graph Neural Networks
D. Köhler, S. Heindorf, ArXiv:2405.12654 (2024).
Causal Question Answering with Reinforcement Learning
L. Blübaum, S. Heindorf, in: The World Wide Web Conference (WWW), ACM, n.d., pp. 2204–2215.
Beyond One-Shot Explanations: A Systematic Literature Review of Dialogue-Based XAI Approaches
D. Mindlin, F. Beer, L.N. Sieger, S. Heindorf, P. Cimiano, E. Esposito, A.-C. Ngonga Ngomo, (2024).
EDGE: Evaluation Framework for Logical vs. Subgraph Explanations for Node Classifiers on Knowledge Graphs
R. Sapkota, D. Köhler, S. Heindorf, in: Proceedings of the 33rd ACM International Conference on Information and Knowledge Management (CIKM ’24), ACM, Boise, Idaho, USA, 2024.
Show all publications
Teaching
Current Courses
- Proseminar: Graph Machine Learning
- Project Group: Explaining Graph Neural Networks (in English)