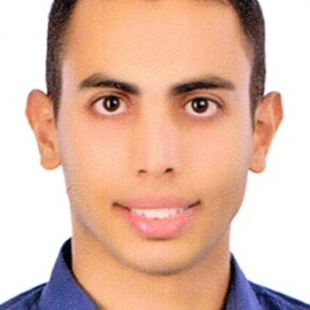
- E-Mail:
- haitham.afifi@uni-paderborn.de
- Phone:
- +49 5251 60-5373
- Web:
- Homepage
- Office Address:
-
Pohlweg 51
33098 Paderborn - Room:
- O3.164
- Office hours:
Tues 12:30 - 13:30
or feel free to passby
Publications
Latest Publications
H. Afifi, F.J. Sauer, H. Karl, in: 2021 IEEE International Conference on Advanced Networks and Telecommunications Systems (ANTS) (ANTS’21), Hyderabad, India, 2021.
H. Afifi, M. Guenther, A. Brendel, H. Karl, W. Kellermann, in: 14. ITG Conference on Speech Communication (ITG 2021), 2021.
M. Gunther, H. Afifi, A. Brendel, H. Karl, W. Kellermann, in: ICASSP 2021 - 2021 IEEE International Conference on Acoustics, Speech and Signal Processing (ICASSP), 2021.
H. Afifi, A. Ramaswamy, H. Karl, in: 2021 IEEE International Conference on Communications (ICC): IoT and Sensor Networks Symposium (IEEE ICC’21 - IoTSN Symposium), Montreal, Canada, 2021.
H. Afifi, A. Ramaswamy, H. Karl, in: 2021 IEEE 18th Annual Consumer Communications \& Networking Conference (CCNC) (CCNC 2021), 2021.
Show all publications
Further Information
Projects
Currently, I am involved in Project: Acousitic Sensor Networks
Research Interests
My current research focuses on wireless networks in the following areas:
- Network optimization and Resource allocation
- Wireless Network Virtualization
- Reinforcement learning
- Wireless MAC protocols for adhoc networks
Currently, I am involved in Project: Acousitic Sensor Networks
Student Theses and Projects
Student Thesis:
- Bachelor (2017): Topology discovery in wireless ad hoc networks
- Bachelor (2018): Task Placement in a Wireless Acoustic Sensor Network using a Genetic Algorithms
- Bachelor (2019): Wireless Virtual Network Embedding using Reinforcement Learning
- Master (2019): Distributed Virtual Network Embedding for Wireless Multi-Hop Networks
Student Projects:
- Team project (SS2017): Distributing Acoustic Functions in Wiereless Sensor Networks
- Team project (SS2018): WiNe for monitoring wireless adhoc networks
- Team project (WS2019): FISSION -- Failover in wIreleSS dIstributed cOmputiNg
If you are intersted in doing your thesis in wireless related topics, please check the current open thesis or feel free to drop me an Email to discuss your interests.