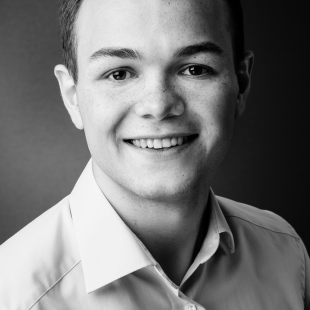
Timon Gurcke
Computational Social Science
Former
- E-Mail:
- timon.gurcke@uni-paderborn.de
- Office Address:
-
Zukunftsmeile 2
33102 Paderborn - Room:
- ZM2.A.03.04
Publications
Latest Publications
M. Alshomary, R. El Baff, T. Gurcke, H. Wachsmuth, in: Proceedings of the 60th Annual Meeting of the Association for Computational Linguistics, 2022, pp. 8782–8797.
M. Alshomary, T. Gurcke, S. Syed, P. Heinisch, M. Spliethöver, P. Cimiano, M. Potthast, H. Wachsmuth, in: Proceedings of the 8th Workshop on Argument Mining, 2021, pp. 184–189.
T. Gurcke, M. Alshomary, H. Wachsmuth, in: Proceedings of the 8th Workshop on Argument Mining, 2021, pp. 67–77.
M. Alshomary, W.-F. Chen, T. Gurcke, H. Wachsmuth, in: Proceedings of the 16th Conference of the European Chapter of the Association for Computational Linguistics: Main Volume, Association for Computational Linguistics, 2021, pp. 224–223.
Show all publications