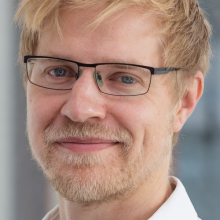
Prof. Dr. Henning Wachsmuth
- E-Mail:
- h.wachsmuth@ai.uni-hannover.de
- Phone:
- +49 511 762 12377
- Web:
- Homepage (Extern)
Computational Social Science
Head - Former
Computational Social Science
- E-Mail:
- henningw@upb.de
- Phone:
- +49 5251 60-6844
- Web:
- Homepage
- Office Address:
-
Zukunftsmeile 2
33102 Paderborn - Room:
- ZM2.A.03.02
Publications
Latest Publications
Identifying Feedback Types to Augment Feedback Comment Generation
M. Stahl, H. Wachsmuth, in: Proceedings of the 16th International Natural Language Generation Conference, n.d.
On-The-Fly Computing -- Individualized IT-services in dynamic markets
C.-J. Haake, F. Meyer auf der Heide, M. Platzner, H. Wachsmuth, H. Wehrheim, On-The-Fly Computing -- Individualized IT-Services in Dynamic Markets, Heinz Nixdorf Institut, Universität Paderborn, Paderborn, 2023.
The Moral Debater: A Study on the Computational Generation of Morally Framed Arguments
M. Alshomary, R. El Baff, T. Gurcke, H. Wachsmuth, in: Proceedings of the 60th Annual Meeting of the Association for Computational Linguistics, 2022, pp. 8782–8797.
"Mama Always Had a Way of Explaining Things So I Could Understand": A Dialogue Corpus for Learning How to Explain
H. Wachsmuth, M. Alshomary, in: Proceedings of the 29th International Conference on Computational Linguistics, 2022, pp. 344–354.
On the Role of Knowledge in Computational Argumentation
Show all publications
A. Lauscher, H. Wachsmuth, I. Gurevych, G. Glavaš, Transactions of the Association for Computational Linguistics (2022).